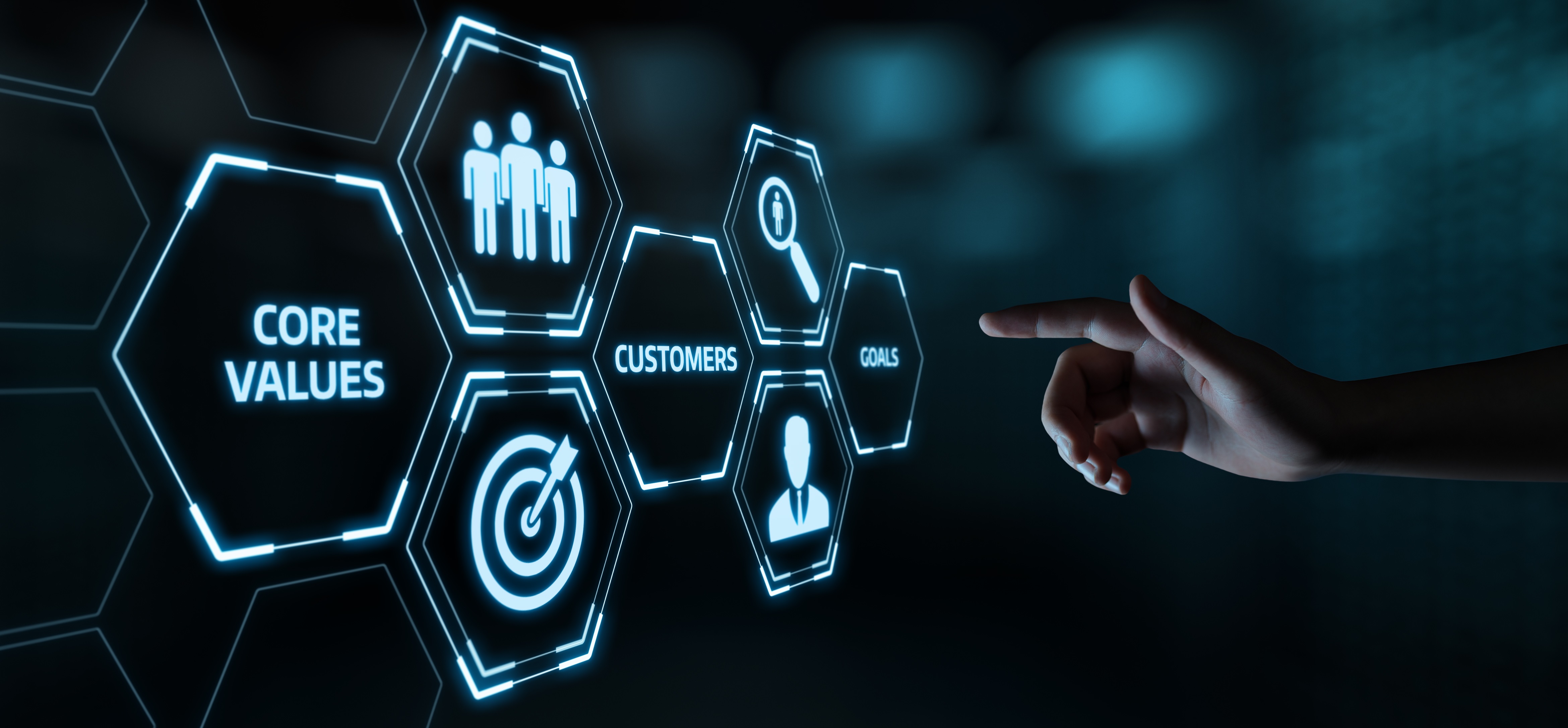
The New Human Role: Teaching AI Our Ways
The New Human Role: Teaching AI Our Ways
Remember when we used to say "it's not rocket science"? Well, nowadays it might be more accurate to say "it's not parameter setting." Because that's what all our jobs are becoming – we're all turning into AI trainers, whether we realize it or not.
The Great Shift in Human Work
Let's face it: AI is rapidly becoming the new rocket scientist, the new chef, the new everything. It's learning to navigate our world with stunning speed, picking up our tools and techniques like a prodigy child who's somehow speedrunning life. But here's the twist – it needs us more than ever, just not in the ways we're used to.
Why We're All Becoming AI Trainers
Think of AI as an incredibly talented foreign exchange student who's just landed in your hometown. Sure, they can read all the maps, learn the street names, and figure out how to use the subway. But what they really need is someone to tell them which food truck has the best tacos, which shortcuts to avoid at night, and why we all collectively pretend not to see each other in elevators.
These unwritten rules, these subtle human preferences – they're the new currency in the AI age. And guess what? We're all experts in being human (well, most days).
The Observable vs. The Invisible
AI is getting remarkably good at learning from our external behaviors. It can watch how we drive, how we write emails, how we design websites. But there's a catch – it can't read our minds (en masse). It can't understand:
-
Why we sometimes choose the longer route home just because it's prettier
-
Why we prefer one font over another for our presentations
-
Why some jokes are okay in certain contexts but not in others
This is where we come in. Our new job is to be the translators of human experience, the codifiers of human preference, the explainers of the inexplicable.
The Industrial Revolution in AI Training
This transformation isn't just happening at an individual level – it's revolutionizing entire industries. Take the semiconductor industry, for example. As Christopher Nguyen, CEO of Aitomatic, points out in his recent talk, they're pioneering a fascinating approach to AI development. Instead of relying solely on generic AI models that know "a lot about many things but lack domain-specific knowledge," they're actively capturing the expertise of veterans with decades of experience.
Think of these amazing LLMs as fresh PhDs. They're amazing in and of themselves, but they don't have domain-specific knowledge and they don't have the experience. He contrasts how a fresh PhD might explain all possible uses of dry chlorosilane in semiconductors, while someone with 20 years of experience would directly solve the problem: "What you need to do is increase the flow rate to 200 SCCM." [1]
Organizational Knowledge Transfer
This principle extends beyond individual expertise to organizational knowledge. Companies are increasingly recognizing the need to codify their collective wisdom, preferences, and protocols. Just as individuals have their quirks and preferences, organizations have their own:
-
Standard Operating Procedures: The "way we do things here" that often goes beyond official documentation
-
Corporate Culture: Unwritten rules about communication styles, decision-making processes, and workplace norms
-
Industry-Specific Knowledge: Specialized expertise that comes from years of collective experience
-
Risk Tolerances: Understanding of what risks are acceptable and which aren't in specific contexts
-
Quality Standards: Both explicit and implicit expectations about what constitutes good work
As Nguyen emphasizes, this knowledge capture is becoming crucial, especially with experienced workers approaching retirement. Companies are racing to develop systems that can "capture the knowledge of somebody who is actually about to retire" and transform it into operational expertise that AI can understand and apply.
Teaching Through Examples
Remember how you learned what was socially acceptable? Probably through a mix of explicit rules ("don't chew with your mouth open") and countless subtle cues. AI needs the same kind of education, but more structured and intentional.
We need to provide:
-
Clear examples of what works and what doesn't
-
Context for why certain choices are better than others
-
Explanations of the nuanced differences between similar situations
-
Real-world scenarios that illustrate our values in action
The Hidden Complexity of Human Knowledge
Here's the beautiful irony: in teaching AI about our world, we're forced to examine and articulate things we've always taken for granted. Why do we prefer certain designs? What makes a conversation feel natural? What are the unspoken rules that guide our social interactions?
It's like trying to explain to someone why water feels wet – it forces us to think deeply about things we've always just known.
The Path to True Alignment
Here's where things get really interesting. When we talk about "AI alignment with human values," we often forget one crucial detail: humanity isn't a monolith. There's no single "Humanity™" that can provide a unified set of preferences and values. Instead, there are billions of us, each with our own perspectives, values, and ways of moving through the world.
This diversity isn't a bug – it's a feature. Just as we've learned to navigate a world full of different viewpoints and preferences, AI needs to learn from each of us individually. It needs to understand not just what "humans want" in some abstract sense, but what you want, what I want, what each of us values and prefers.
Learning from Our Collective Wisdom
One way we already express our collective preferences is through laws and regulations. Think about it: most humans prefer not to be murdered, so we have laws against murder. We prefer not to drink poison, so we have food and drug safety regulations. We prefer to breathe clean air, so we have environmental protection laws.
These aren't arbitrary rules – they're codified expressions of our shared preferences, emerged from centuries of human experience and negotiation. For AI to truly serve humanity, it needs to understand both these formal expressions of our collective will and the informal, personal preferences that guide our daily lives.
Taking Back Control of the Algorithm
Here's perhaps the most exciting part: this new paradigm offers us a chance to reclaim control over the algorithms that increasingly shape our lives. Until now, most AI systems have been trained primarily on our observable actions and behaviors – what we click, what we buy, where we go. But behavior doesn't always reflect preference.
Maybe you click on clickbait headlines even though you'd prefer more substantive news. Maybe you scroll social media longer than you'd like because it's designed to be addictive. Maybe you have lost someone close to you because the algorithms pulled you each into your own information silo.
By actively participating in AI training and parameter setting, we have the opportunity to teach AI systems not just what we do, but what we truly want and value. It's a chance to inject human wisdom, preference, and nuance into systems that have previously only seen our surface-level behaviors.
The Road Ahead
So perhaps our collective job description needs one more bullet point:
Position: Human Experience Translator
-
Key Responsibilities: Articulating the ineffable, codifying the intuitive, teaching machines the art of being human
-
Required Skills: Being thoughtfully human and deliberately explainable in one's actions and decisions
-
Critical Mission: Ensuring AI understands not just what we do, but what we prefer, value, and aspire to be
The future of AI isn't just about making machines smarter – it's about making them wiser. And wisdom, as we've learned through millennia of human experience, comes from understanding not just actions, but intentions; not just behaviors, but values; not just what is, but what ought to be.
Remember: AI can learn to play our instruments and even compose new music, but we're the ones who need to teach it why music moves us in the first place. That's not just a job – it's a calling for our times.
References
[1] Nguyen, C. (2024, October). "Industrial AI and Domain Expertise in Semiconductor Manufacturing." Speech presented at Stanford’s Industrial AI Conference. https://www.youtube.com/watch?v=aWEaEgV1pHQ Quotes and insights from sections:
-
Comparison of generic LLMs to "fresh PhDs" versus experienced practitioners
-
Discussion of domain expertise capture in semiconductor manufacturing
-
Analysis of knowledge transfer from retiring experts to AI systems
-
Examples of practical problem-solving in semiconductor processes
Posts
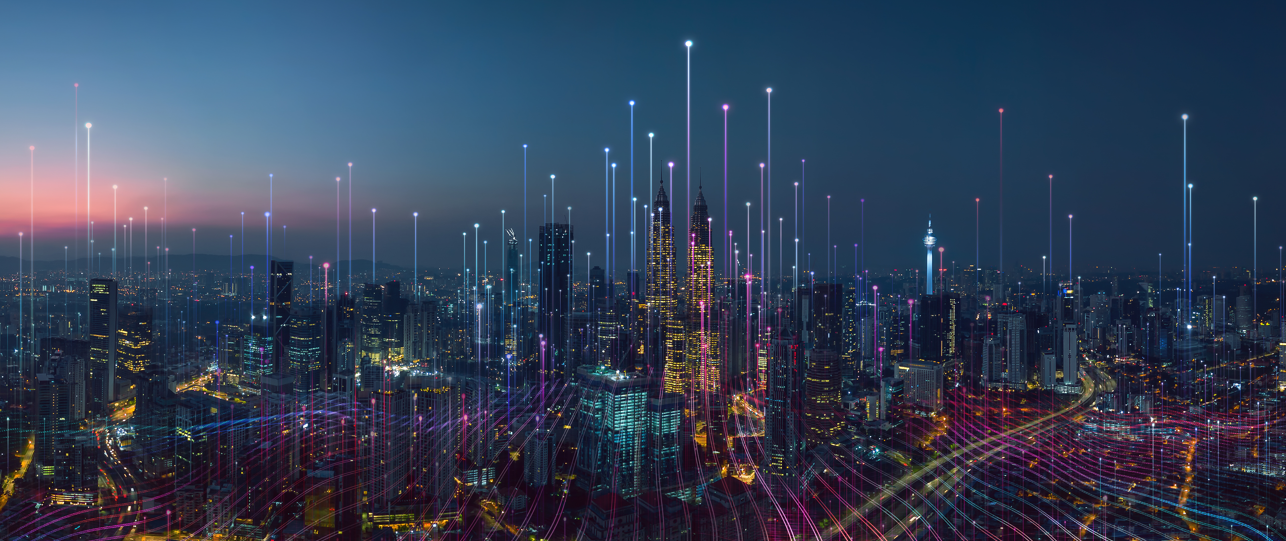
AI and Ethics in Practice: Podcast Episode
Our CEO Dr. Ganesan Keerthivasan and our Head of AI Ethics Dr. Tom Tirpak recent....
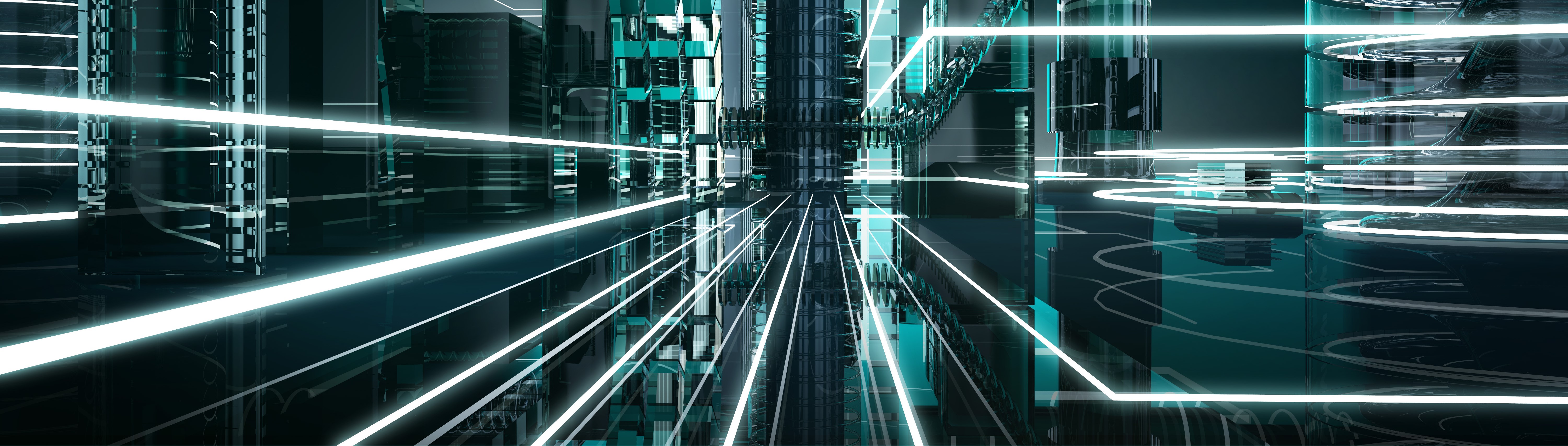
Why MLOps is Required: The Mission Control Imperative
Taking to heart the advice of business leadership expert Simon Sinek, let's star....
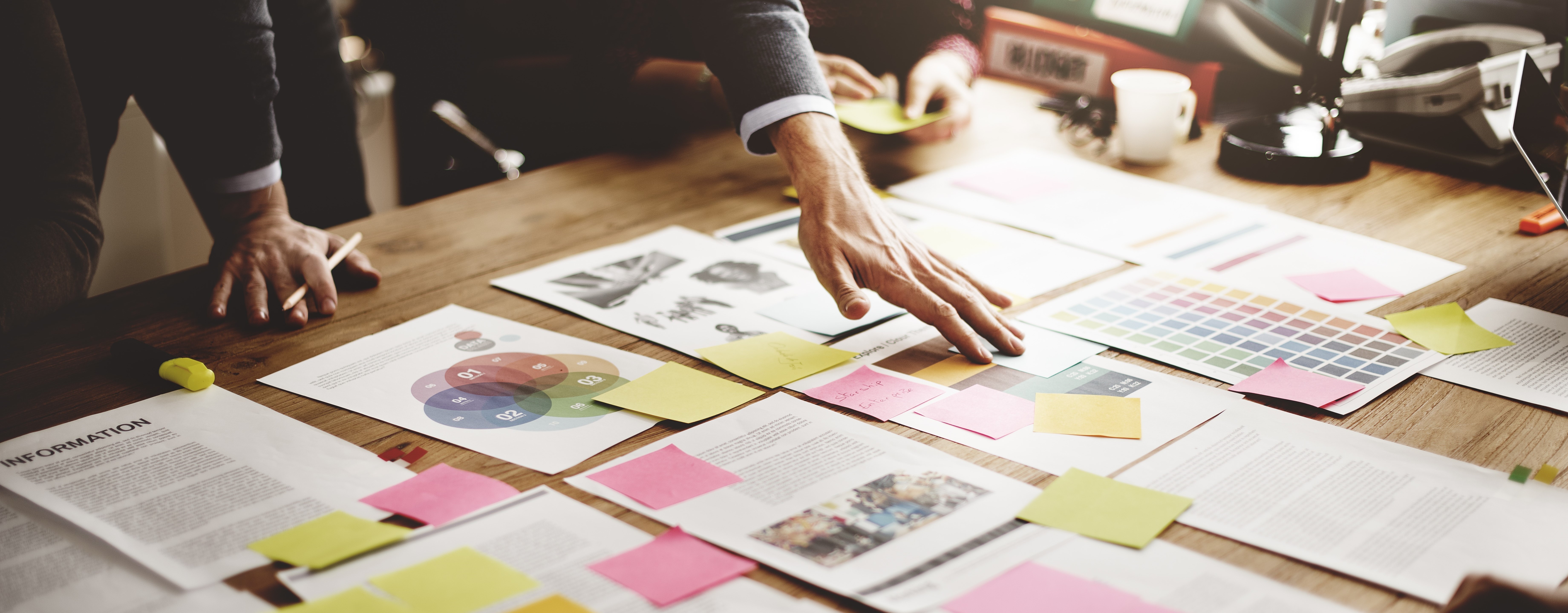
AI System Inventories - The Foundation for Governance
In a conference room last week, a CTO asked her team a seemingly simple question....
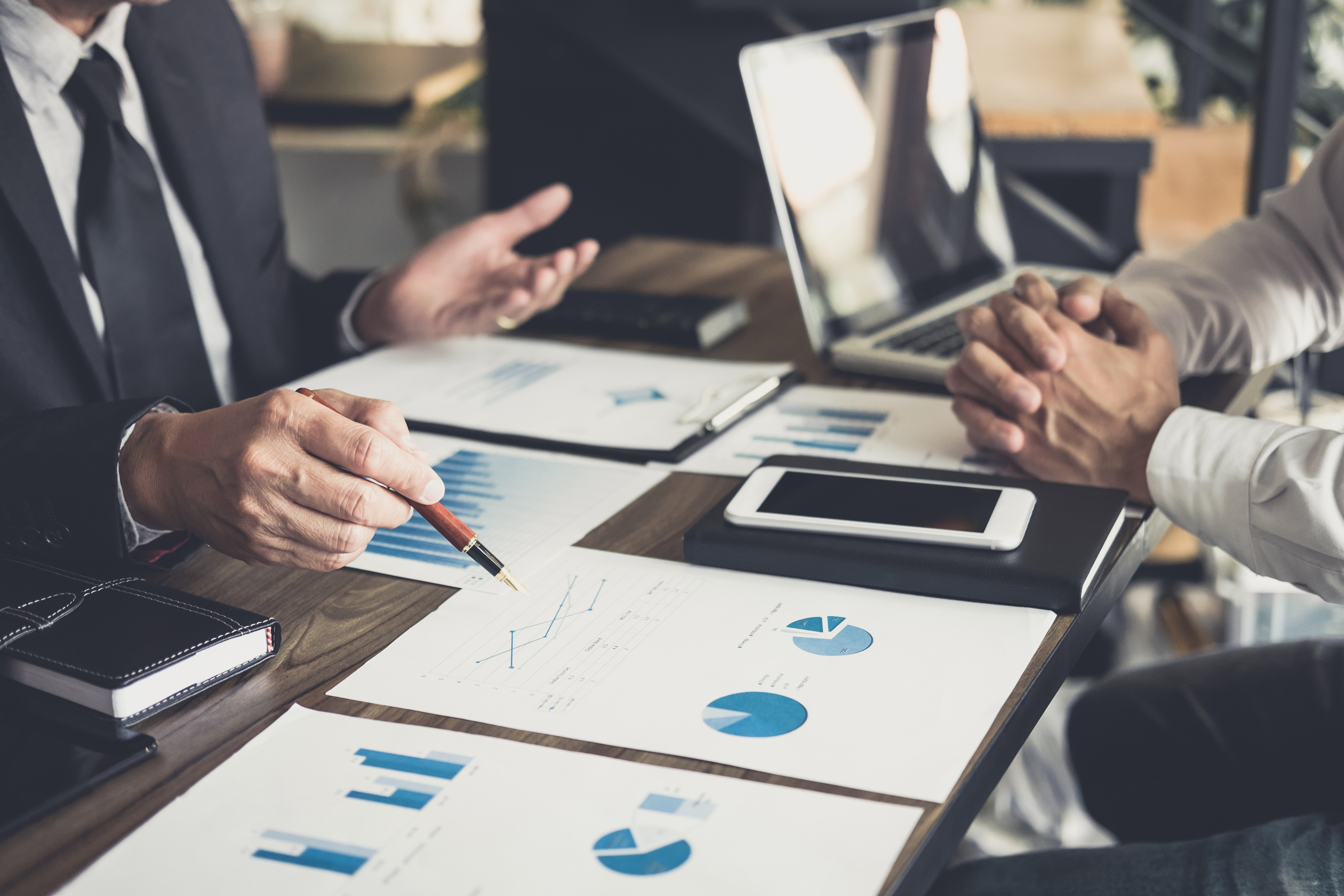
The Legal Implications of Ethical AI
As AI continues to permeate business and society, the legal landscape surroundin....
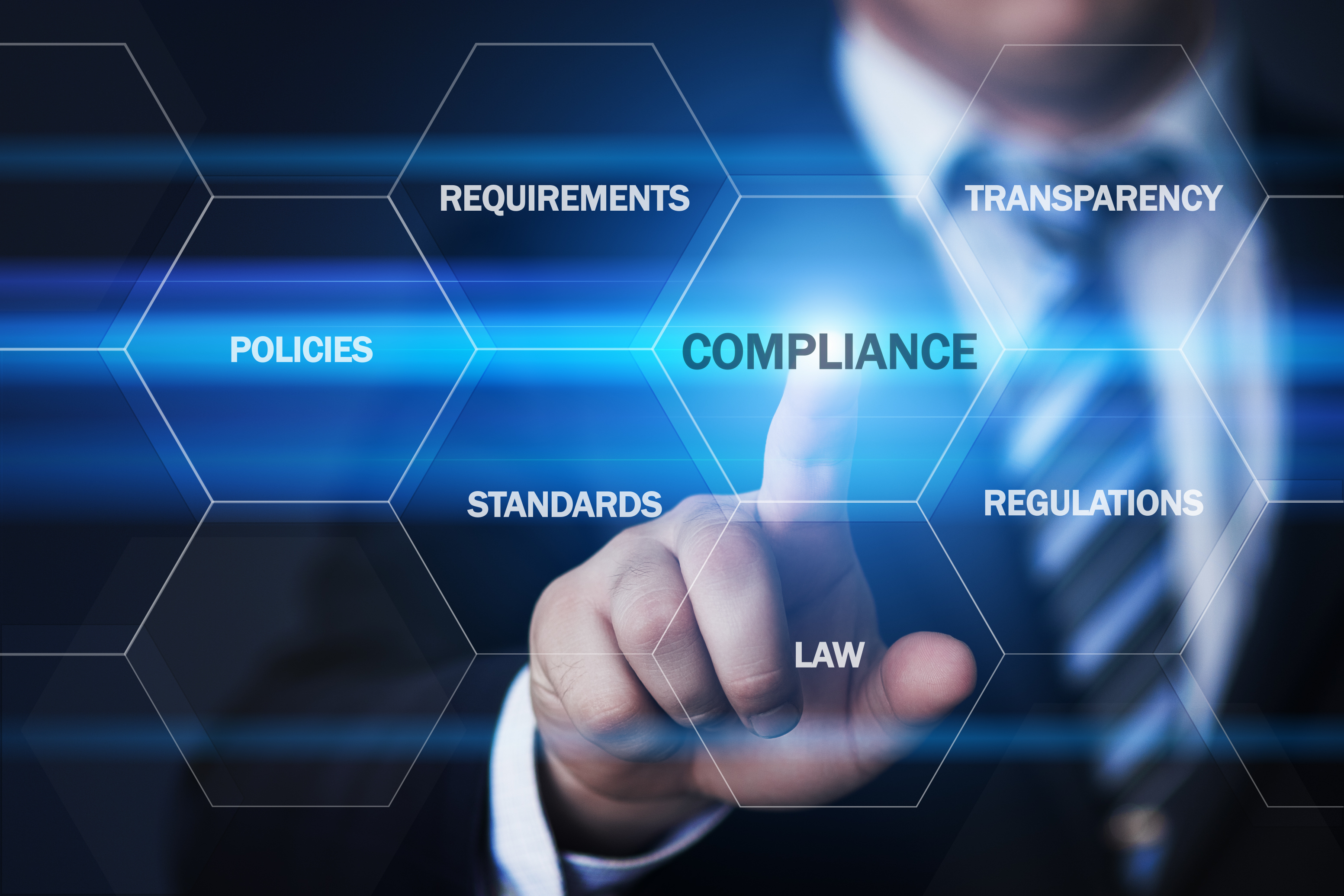
Installing AI Governance
AI Governance: Installing a framework in your business In the rapidly evolving ....
-min.jpg)
Ensuring Ethical AI: The Value of Third-Party Assessments
In the rapidly evolving landscape of artificial intelligence, organizations face....