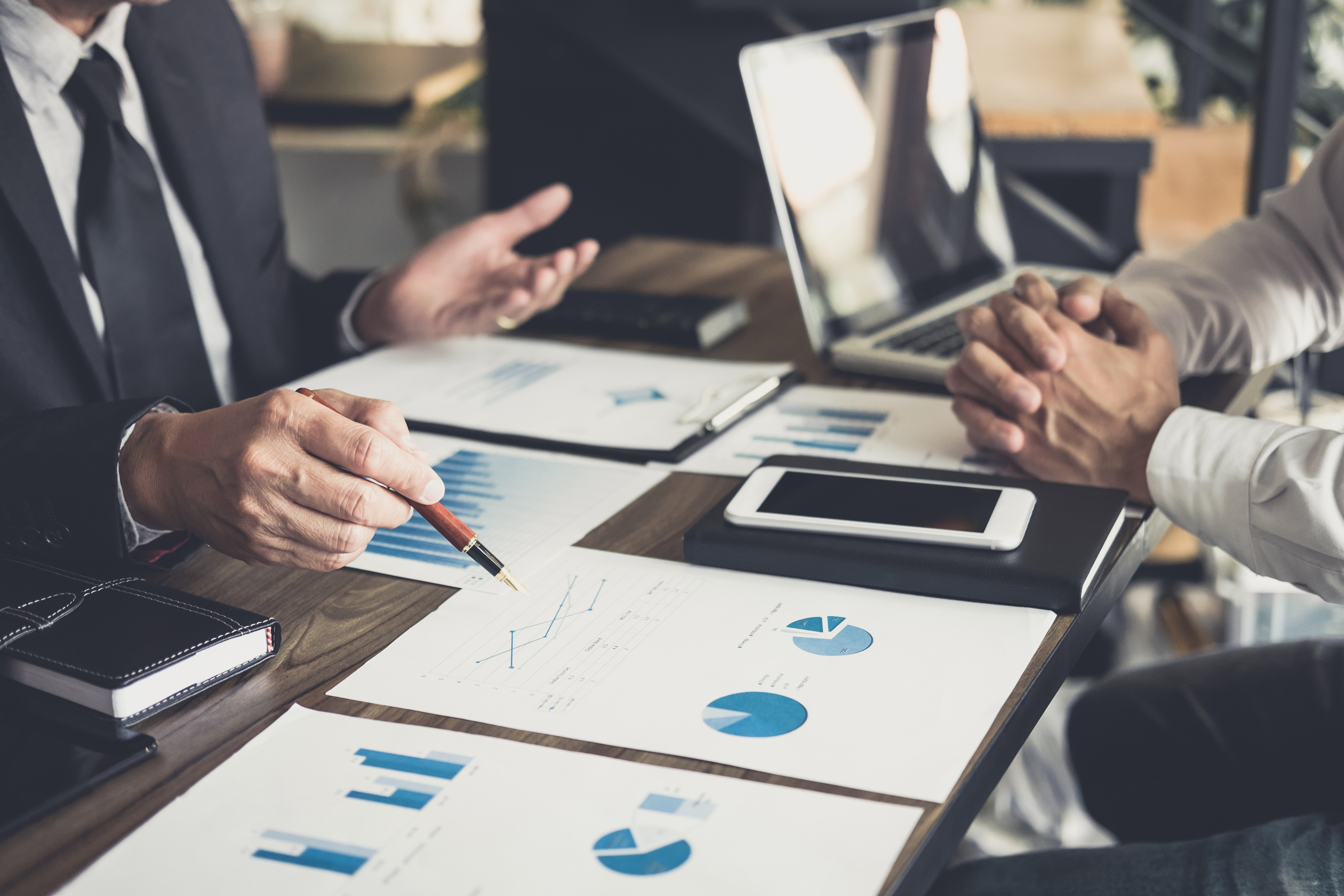
The Legal Implications of Ethical AI
As AI continues to permeate business and society, the legal landscape surrounding its implementation is rapidly evolving. Recent years have seen an increase in litigation related to AI, highlighting the critical importance of adhering to established ethical standards. While ethics may be construed as a broad philosophical topic, standards setting organizations like IEEE have more narrowly defined the scope for Ethical AI (please refer to our prior blog for more detail).
The DAIL1 (Database of AI Litigation) reports over 200 legal cases pertaining to artificial intelligence and machine learning. Trends through 2023 suggest plaintiffs are shifting focus from targeting AI developers to holding firms that purchase and implement AI solutions accountable.2 Fines and settlements in each of these cases can total in the millions of dollars3, excluding reputational damage and required system overhauls.
IEEE CertifAIEdTM is a certification program for assessing AI ethics. The program defines Ethical AI in terms of accountability, transparency, algorithm bias, and privacy. Each of these buckets addresses litigation risk as explained below.
Accountability: Assigning Responsibility for AI Outcomes
As AI systems become more autonomous in decision-making, assigning responsibility for their outcomes becomes increasingly complex and legally significant. As organizations rapidly adopt this evolving technology, governance and human oversight are particularly important. “Accountability” in an ethics context is defined as a willingness to take responsibility for actions, decisions, and their consequences.4
Case Study: DOJ and States v. RealPage5
This antitrust lawsuit alleges that RealPage's AI-powered software enables rent price-fixing, raising questions about the potential for AI systems to facilitate anti-competitive behavior. The case highlights the challenges in determining liability when AI systems contribute to potentially illegal market practices.
Adherence to Ethical AI standards for accountability could help mitigate such legal risks by:
-
Establishing clear lines of responsibility for AI system outcomes
-
Implementing robust oversight mechanisms for AI-driven decisions
-
Ensuring human review of high-risk AI recommendations, especially in sensitive areas like pricing
By implementing these measures, companies can demonstrate due diligence and potentially limit their liability in cases where AI systems contribute to unintended consequences.
Transparency and Explainability: Ensuring AI Decisions are Understandable
Insufficient visibility into AI models has led to legal challenges, particularly when AI decision-making processes are opaque. “Transparency and Explainability” in an ethics context is defined as appropriate openness, clarity, and ability to understand and interpret key processes, requiring users and vendors of AI to be sufficiently aware of their systems' capabilities and limitations.6
Case Study: FTC v. DoNotPay7
In this case, the Federal Trade Commission took action against DoNotPay, a company claiming to offer AI-powered legal services that failed to deliver on its promises. The company marketed its AI as capable of drafting complex legal documents and providing personalized legal advice, but users reported receiving generic, sometimes incorrect information. DoNotPay agreed to pay a $193,000 settlement, notify customers of their product’s limitations, and adhere to stricter guidelines for its marketing going forward.
This case demonstrates the increased regulatory scrutiny of AI marketing claims and the importance of transparency in AI capabilities. Adherence to Ethical AI standards for transparency and explainability could have mitigated these issues by:
-
Requiring clear and appropriately accessible documentation of the technology’s capabilities, limitations, and system design
-
Establishing regular audits to verify AI performance claims post product launch
-
Ensuring AI systems’ capabilities are presented accurately to users
By following these practices, companies can build trust with users and regulators, reducing the risk of legal action stemming from misrepresented AI capabilities.
Acceptable Algorithmic Bias and Fairness: Unintended Biases and Harm to Specific Groups
AI systems that exhibit bias or discrimination are increasingly facing legal challenges, particularly in domains like employment and lending. “Acceptable Bias and Fairness” in an ethics context refers to establishing boundaries of acceptance in unintended bias, to minimize negative impacts to individuals, communities, and society.8
Key in this definition is the concept of acceptable bias. Biases may naturally occur in AI development, but an ethically aligned process will correct emerging or detected bias through risk management, design changes, and compensation mechanisms.8 Algorithm bias that leads to outcomes which differ significantly from requirements is unacceptable, while bias within established boundaries is acceptable.
Case Study: Mobley v. Workday9
This class action lawsuit alleges that Workday's AI-powered applicant screening tools discriminate based on race, age, and disability. The court ruling accepts the “agent” theory of liability, setting precedence for AI vendors to face responsibility for employment discrimination claims involving their customers. The case highlights potential bias in AI hiring systems and the legal risks associated with using AI in employment decisions.
Adherence to Ethical AI standards for fairness and bias mitigation could help prevent such legal issues by:
-
Assessing stakeholders’ needs; organizations should identify all relevant stakeholders (e.g., customers, partners) and directly consulting them regarding their priorities around algorithmic bias
-
Identifying and correcting biases in systems development, including in constructing training sets
-
Implementing continuous monitoring and evaluation post-launch
-
Establishing clear processes for addressing and correcting biases if/when identified
-
Maintaining human oversight and accountability
By proactively addressing bias concerns, companies can reduce the risk of discrimination lawsuits and contribute to more equitable AI-powered ecosystems.
Privacy: Protecting Sensitive Data
AI systems that violate privacy rights are increasingly subject to legal action, particularly as data protection regulations become more stringent globally. Privacy in an ethics context largely pertains to information privacy and data protection concerns. The ethical definition of privacy generally overlaps with but may be broader than existing legal frameworks, emphasizing individuals’ rights to protect their information from unauthorized access or misuse.10
Case Study: Patel v. Facebook11
In this 2020 case, Facebook (now Meta) announced it would pay $550M to settle a class-action lawsuit alleging violation of the Illinois Biometric Information Privacy Act (BIPA). The class action alleged Facebook collected and stored user facial data without proper consent or notice for its “Tag Suggestion” feature. The feature used facial recognition to suggest users to “tag” others when posting photos. BIPA requires written consent before collecting key biometric data, which the suit alleged Facebook did not obtain.
Adherence to IEEE standards for privacy protection could help mitigate such legal risks by:
-
Adequately informing users about data collection and use.
-
Establishing consent mechanisms for controlling how data are collected and used.
-
Avoiding overreaching algorithms, which draw inferences based upon isolated data points and may cause users a feeling of encroachment on their privacy.
Conclusion
The legal implications of Ethical AI are becoming increasingly significant as courts, regulators, and the public demand greater accountability for AI-driven decisions and outcomes. By proactively addressing ethical concerns and adhering to established standards like those set by IEEE, organizations can not only mitigate legal risks but also build trust with customers and stakeholders.
As the AI landscape evolves, staying ahead of ethical considerations and aligning with new and existing AI-related laws will be crucial for long-term success. As case studies illustrate, algorithm builders and users should also ensure continued compliance with laws that were written without AI in mind, such as those around data consent and discrimination.
ZealStrat is committed to leading the charge in this crucial endeavor. Our AI Ethics services are designed to guide organizations in developing, deploying, and managing AI ethically and responsibly. With a team of IEEE Authorized Assessors, we offer comprehensive services, including AI Ethics Bootcamps, AI Risk Profile Creation, Documentation Discovery, Playbook Creation, and Preparation for IEEE Certification. We provide ongoing support to help clients maintain their ethical AI certifications and build trust with stakeholders. Rooted in industry standards and best practices, our approach helps organizations navigate the complex regulatory landscape, ensure compliance, and foster a culture of ethical AI governance.
Partner with ZealStrat to secure your place at the forefront of ethical AI innovation. For more information, contact us at contact@zealstrat.com.
References
1 George Washington University. (n.d.). AI litigation database. The George Washington University Law School. Retrieved November 6, 2024, from https://blogs.gwu.edu/law-eti/ai-litigation-database/
2 K&L Gates. (2023, September 5). Recent trends in generative artificial intelligence litigation in the United States. K&L Gates LLP. Retrieved November 6, 2024, from https://www.klgates.com/Recent-Trends-in-Generative-Artificial-Intelligence-Litigation-in-the-United-States-9-5-2023
3 Holistic AI. (2023, October 10). The high cost of non-compliance: Penalties under AI law. Holistic AI. Retrieved November 6, 2024, from https://www.holisticai.com/blog/high-cost-non-compliance-penalties-under-ai-law
4 IEEE CertifAIEd. (2022). Ontological specification for ethical accountability. IEEE Standards Association.
5 Department of Justice. (2024). DOJ and States File Antitrust Lawsuit Against RealPage for AI-Enabled Price Fixing. DOJ News.
6 IEEE CertifAIEd. (2022). Ontological specification for ethical transparency. IEEE Standards Association.
7 Federal Trade Commission. (2024). FTC Takes Action Against DoNotPay for Deceptive AI Claims. FTC Press Releases.
8 IEEE CertifAIEd. (2022). Ontological specification for ethical algorithmic bias. IEEE Standards Association
9 Mobley v. Workday, Inc., No. 3:23-cv-04607 (N.D. Cal. 2023).
10 IEEE CertifAIEd. (2022). Ontological specification for ethical privacy. IEEE Standards Association.
11 Patel v. Facebook: Facebook Settles Illinois Biometric Information Privacy Act (BIPA) Violation Suit. (2020). Harvard Journal of Law & Technology Digest.
Posts
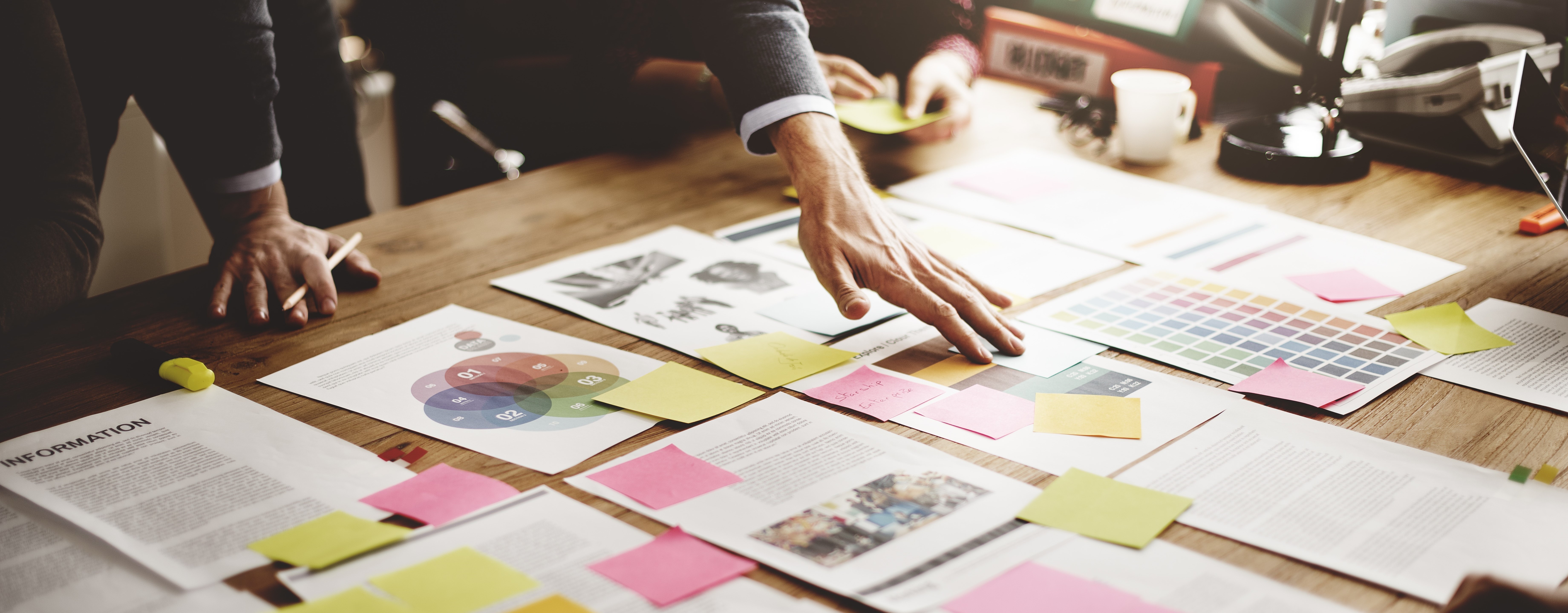
AI System Inventories - The Foundation for Governance
In a conference room last week, a CTO asked her team a seemingly simple question....
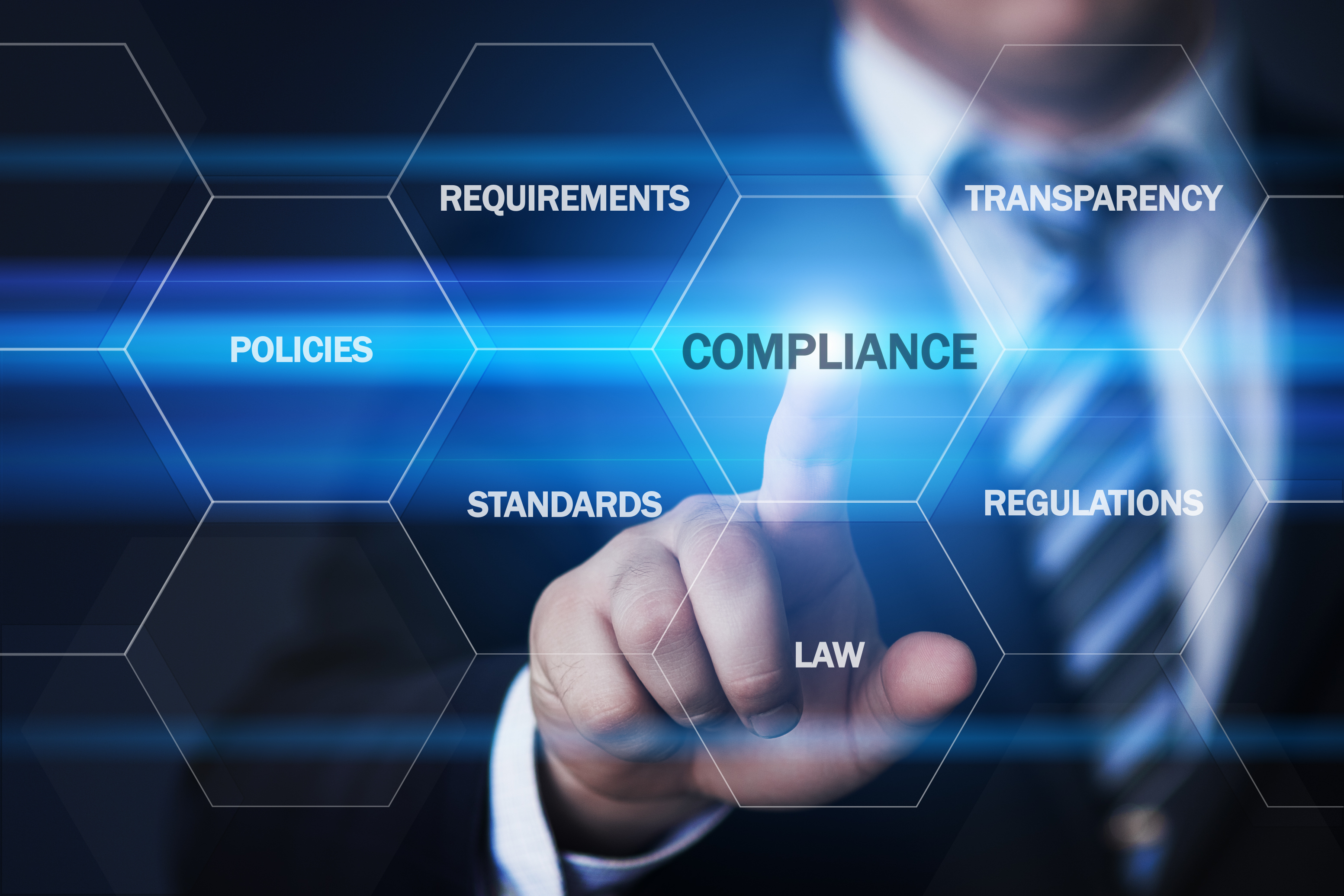
Installing AI Governance
AI Governance: Installing a framework in your business In the rapidly evolving ....
-min.jpg)
Ensuring Ethical AI: The Value of Third-Party Assessments
In the rapidly evolving landscape of artificial intelligence, organizations face....
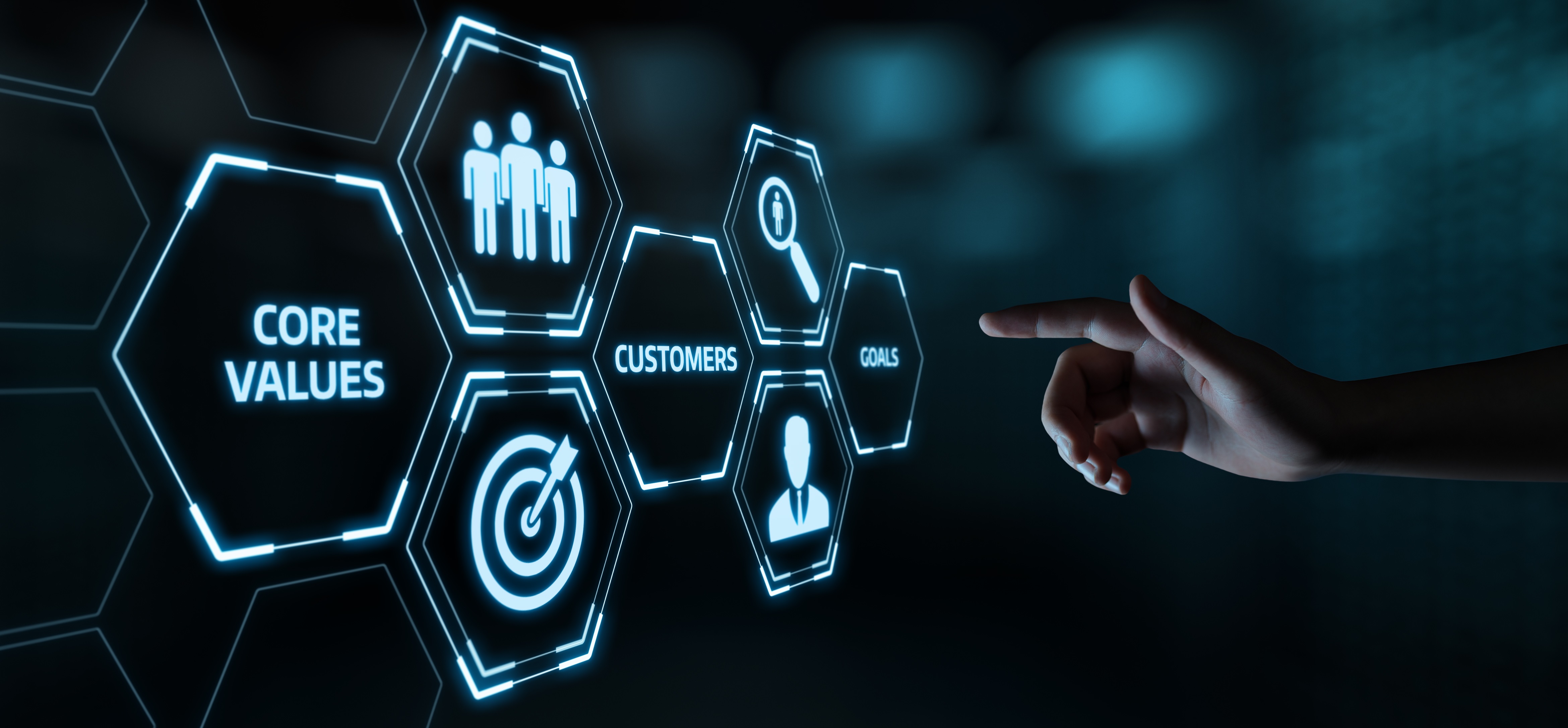
The New Human Role: Teaching AI Our Ways
The New Human Role: Teaching AI Our Ways Remember when we used to say "it's not....
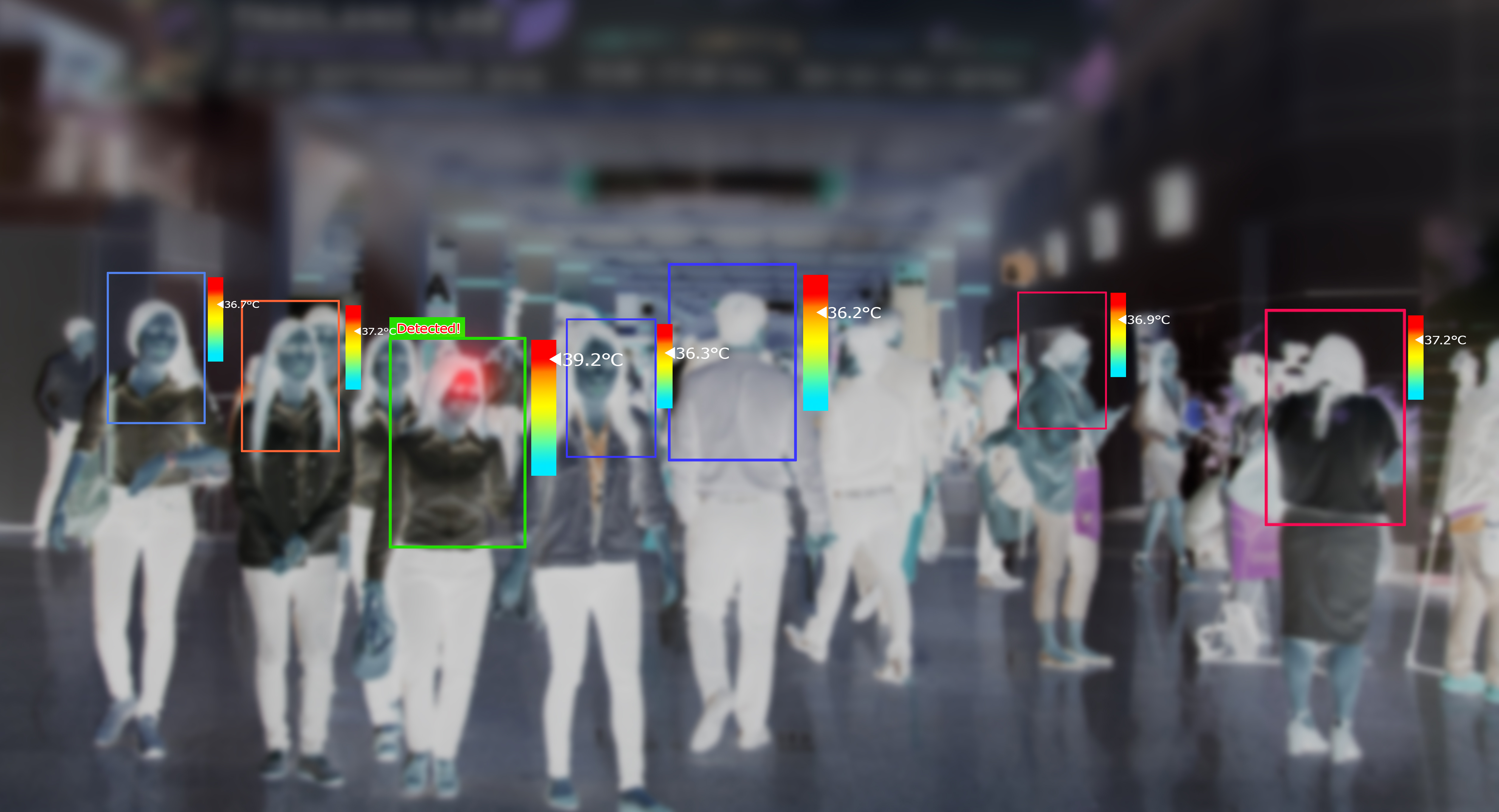
The Imperative of High Ethical Standards for AI in Business
“With great power comes great responsibility”. Derivations of ....