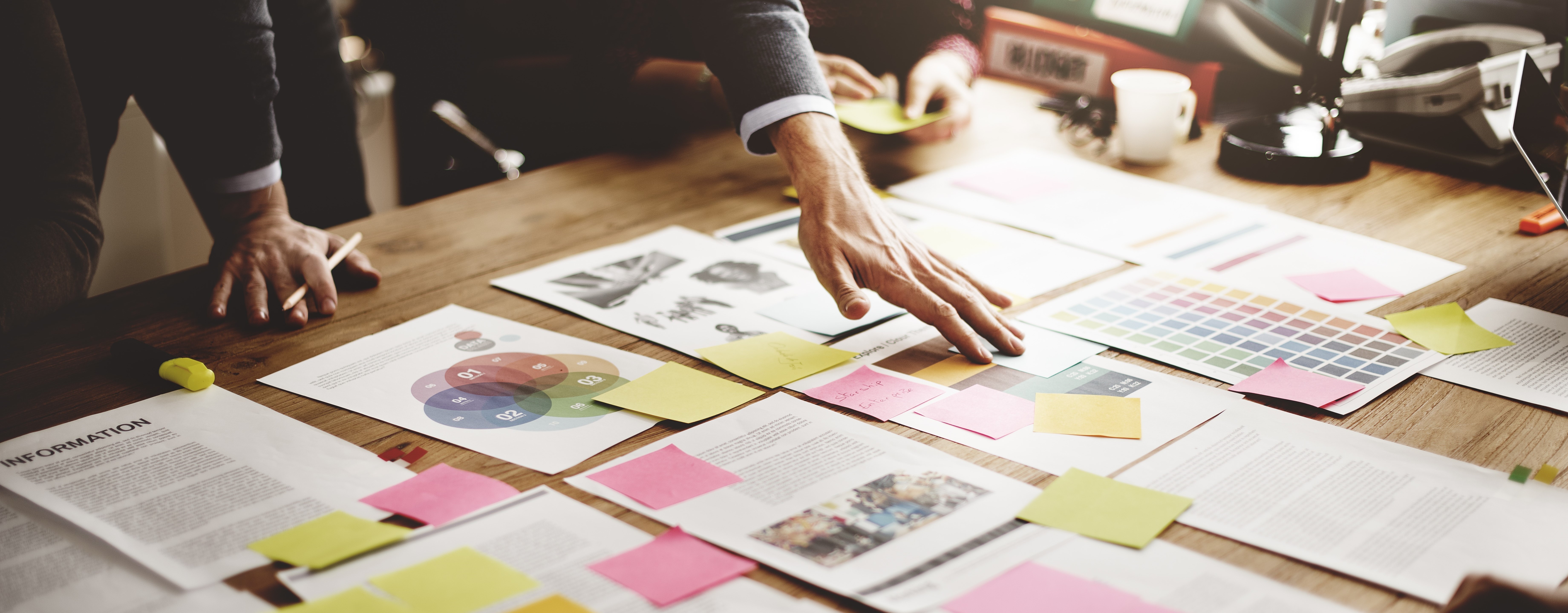
AI System Inventories - The Foundation for Governance
In a conference room last week, a CTO asked her team a seemingly simple question: "How many AI models do we have running in production?" The uncomfortable silence that followed is becoming an all-too-familiar scene in enterprises worldwide. This scenario illustrates a fundamental challenge in AI governance that many organizations are grappling with today.
A Tale of Two Intelligences
When we discuss AI governance, we often make a distinction between human and machine intelligence. Perhaps it's more useful to think in terms of Biological Intelligence and machine Intelligence. Both forms process information, learn from experience, and require consistent oversight. Just as organizations maintain detailed records of their human resources, they need comprehensive inventories of their machine intelligence resources.
The Hidden Value of Knowing What You Have
According to the NIST AI Risk Management Framework Playbook (2023), "An AI system inventory is an organized database of artifacts relating to an AI system or model" (p. 14) [1]. This clinical definition barely scratches the surface of its true value. Think of an AI inventory as your organization's machine intelligence map – it tells you not just what you have, but how everything connects and who's responsible when things go wrong.
The power of a well-maintained AI inventory becomes clear when we look at real-world scenarios. Consider what happens when stakeholders discover that a model has been making unexpected decisions. Without an inventory, teams might spend days just identifying the system's ownership and documentation. With a proper inventory, they can quickly access incident response plans, system documentation, and contact information for the people that maintain the relevant AI system.
From Homebrew to Enterprise: The AI Ecosystem
The reality of enterprise AI includes more than just the headline-grabbing models from companies like Anthropic and OpenAI. Your organization likely has a complex tapestry of AI systems ranging from homegrown models developed for specific use cases to open-source implementations from Hugging Face, alongside enterprise AI services. Each of these comes with its own governance needs and risks.
Managing this diverse ecosystem becomes exponentially more complex as your AI footprint grows. Without proper tracking and governance, organizations often find themselves with redundant systems, orphaned models, and unclear ownership structures. This complexity isn't just an organizational challenge – it's a significant business risk that directly impacts your bottom line.
The True Cost of Not Knowing
When organizations balk at the resource requirements for maintaining an AI inventory, they're often missing the bigger picture. The NIST Playbook emphasizes that organizations should "establish policies that define a specific individual or team that is responsible for maintaining the inventory" (p. 14). This isn't bureaucratic overhead – it's essential risk management. The most significant costs often come not from maintaining an inventory but from not having one. Duplicate investments, compliance violations, and delayed incident responses can far outweigh the investment in proper documentation and tracking. More importantly, as regulatory scrutiny of AI systems increases, having a comprehensive inventory isn't just good practice – it's becoming a necessity.
In July 2024, a significant global IT outage occurred due to a faulty update from CrowdStrike's Falcon Sensor security software, affecting approximately 8.5 million Windows systems worldwide. The widespread impact disrupted various sectors, including airlines, hospitals, and financial institutions. A notable challenge during the recovery process was the difficulty organizations faced in identifying which systems were affected and who was responsible for their maintenance. This lack of clarity led to prolonged downtime and delayed incident responses, highlighting the critical importance of maintaining a comprehensive inventory of IT systems and clearly defining ownership to facilitate swift recovery during such incidents.[2]
What would a little investment in system mapping have saved the world during that outage?
Beyond Documentation: The Evolution of AI Systems
Understanding your AI inventory goes deeper than documentation of the currently deployed systems – it's about understanding the living, growing nature of these systems. Modern AI systems aren't static; they evolve, adapt, and sometimes expand their capabilities in unexpected ways. This organic evolution is precisely why traditional static inventories fall short. An effective inventory must capture not just what a system is, but what it's becoming.
The Missing Piece: Evaluation Frameworks
Perhaps the most overlooked aspect of AI inventories is the evaluation or “evals” framework. Every AI system in your inventory needs a clear differentiation of "good" versus "degraded" performance. Without standardized evaluation metrics, even the most detailed inventory becomes merely a catalog of assets rather than a meaningful governance tool.
The evaluation framework should encompass not just performance metrics, but also action and response parameters, ethical norms, and business impact assessments. This comprehensive approach to evals ensures that your inventory serves as both a technical reference and a strategic management tool. As you build your evaluation framework and tests, make sure you run it as a part of your AI Ops pipeline. This will radically improve your AI governance and system inventory from a static catalog into a dynamic health monitoring system.
Building Your Knowledge Base
Starting your AI inventory journey doesn't have to be overwhelming. The key is to think of your inventory as a living knowledge base rather than a static document. This means integrating it with existing change management processes, establishing regular review cycles, and creating clear protocols for updating and maintaining the information.
The most successful AI inventories grow organically alongside your AI systems, capturing not just technical specifications but also the relationships between systems, their impact on business processes, and their governance requirements. By treating your inventory as a dynamic system rather than a collection of compliance checklists, you create a foundation for effective AI governance.
Looking Forward
As we continue to integrate more machine intelligence into our organizations, the line between human and machine intelligence will become increasingly blurred. The organizations that succeed in this new landscape will be those that can effectively integrate and govern both forms of intelligence. An AI system inventory isn't just a document – it's a living map of your organization's cognitive capabilities.
Just as we wouldn't dream of running a company without knowing our human resources, we can't effectively operate in the age of AI without a clear understanding of our machine intelligence assets. The NIST AI RMF Playbook provides a framework, but it's up to each organization to build and maintain an AI governance system that adequately manages their risk.
The next time someone asks about your organization's AI systems, will you be met with uncomfortable silence, or will you have the answers at your fingertips?
At ZealStrat, we're committed to helping organizations navigate these challenges. Our team of experts can guide you in developing and implementing AI governance frameworks that are both effective and adaptable to your specific needs. Together, we can work towards a future where AI is not just powerful, but also trustworthy and aligned with human values.
Note: This article references the NIST AI Risk Management Framework Playbook (2023), specifically the guidance provided in GOVERN 1.6 regarding AI system inventories.
-------------------
References:
[1] https://www.nist.gov/itl/ai-risk-management-framework/nist-ai-rmf-playbook
[2] https://en.wikipedia.org/wiki/2024_CrowdStrike-related_IT_outages
A Practical Checklist for Building Your AI Inventory
Initial Discovery
□ Department-by-department AI usage survey
□ Identification of automated decision-making processes
□ Mapping of third-party AI service integrations
Core Documentation
□ Model purpose and scope definitions
□ Data source and dependency mapping
□ Risk classification framework
□ Ownership and responsibility assignments
□ Performance metrics and thresholds
Evaluation Framework
□ Baseline performance measurements
□ Test case libraries
□ Evaluation schedules
□ Degradation triggers and thresholds
□ Incident response protocols
Governance Structure
□ Review responsibilities and schedules for high-risk systems
□ Change management integration
□ Stakeholder communication plan
□ Automated monitoring setup
□ Compliance reporting framework
Posts
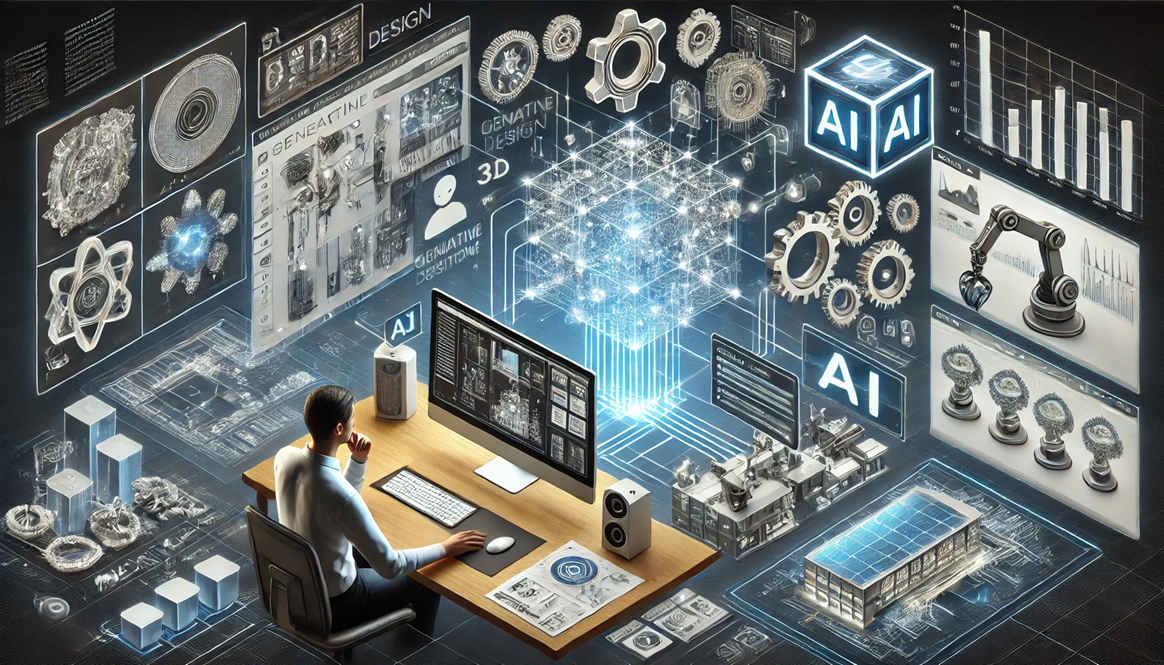
Managing AI Risks in New Product Development
Artificial Intelligence (AI) is rapidly transforming New Product Development (NP....
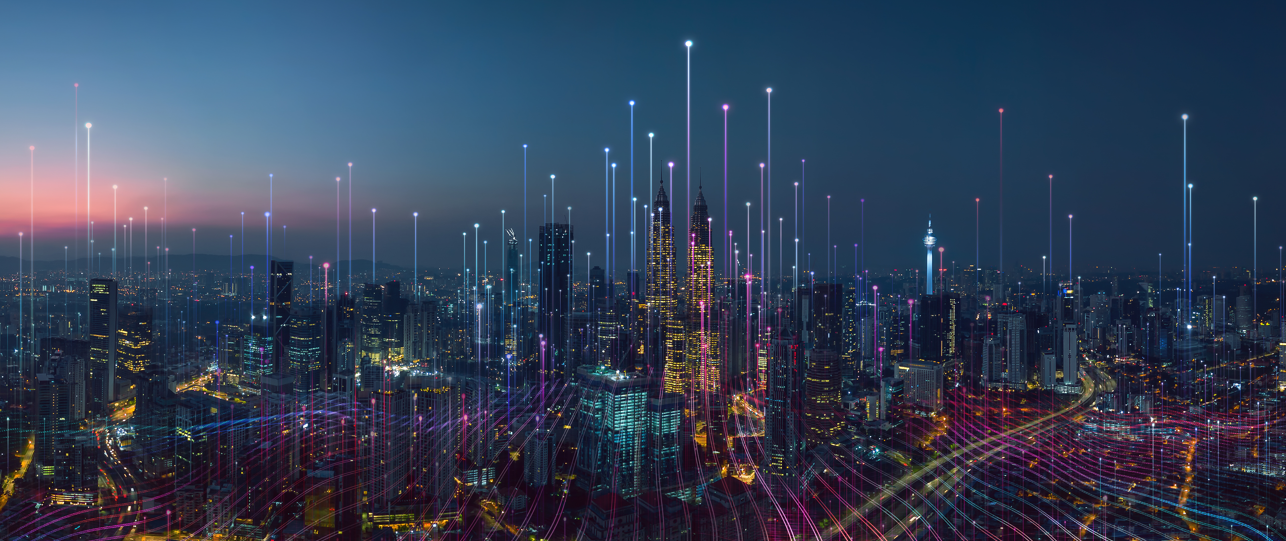
AI and Ethics in Practice: Podcast Episode
Our CEO Dr. Ganesan Keerthivasan and our Head of AI Ethics Dr. Tom Tirpak recent....
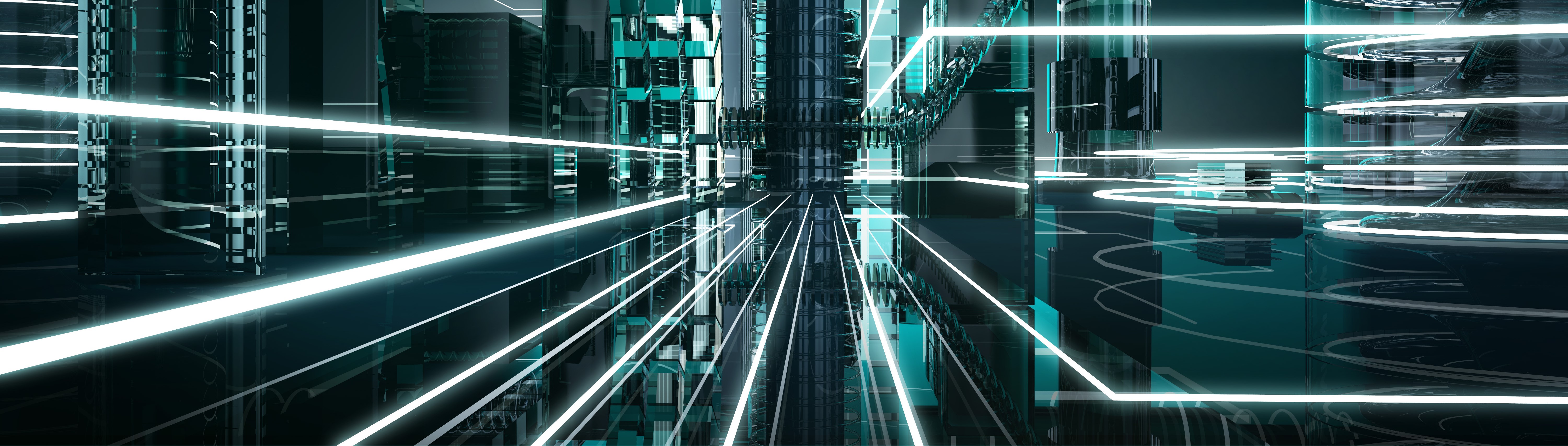
Why MLOps is Required: The Mission Control Imperative
Taking to heart the advice of business leadership expert Simon Sinek, let's star....
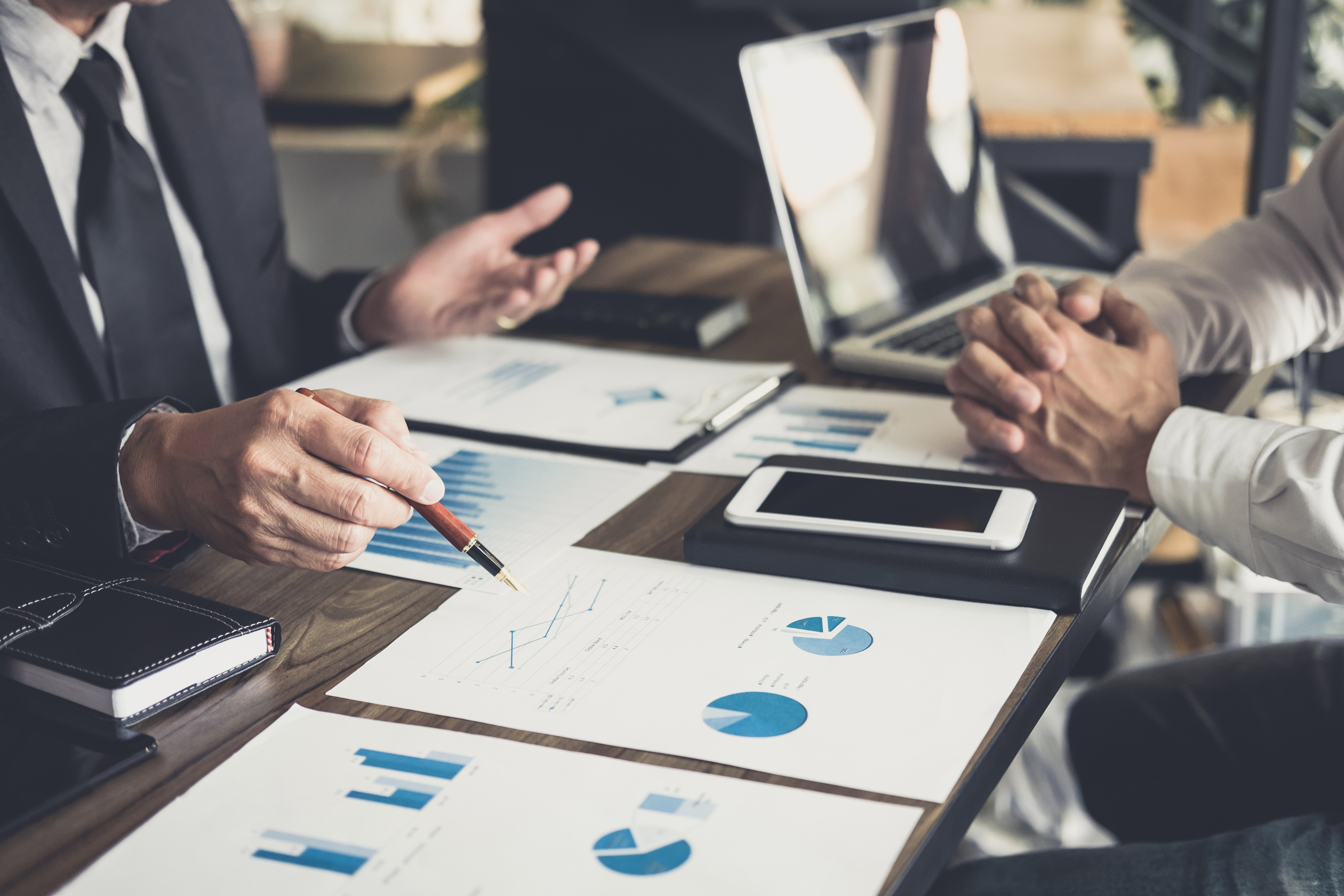
The Legal Implications of Ethical AI
As AI continues to permeate business and society, the legal landscape surroundin....
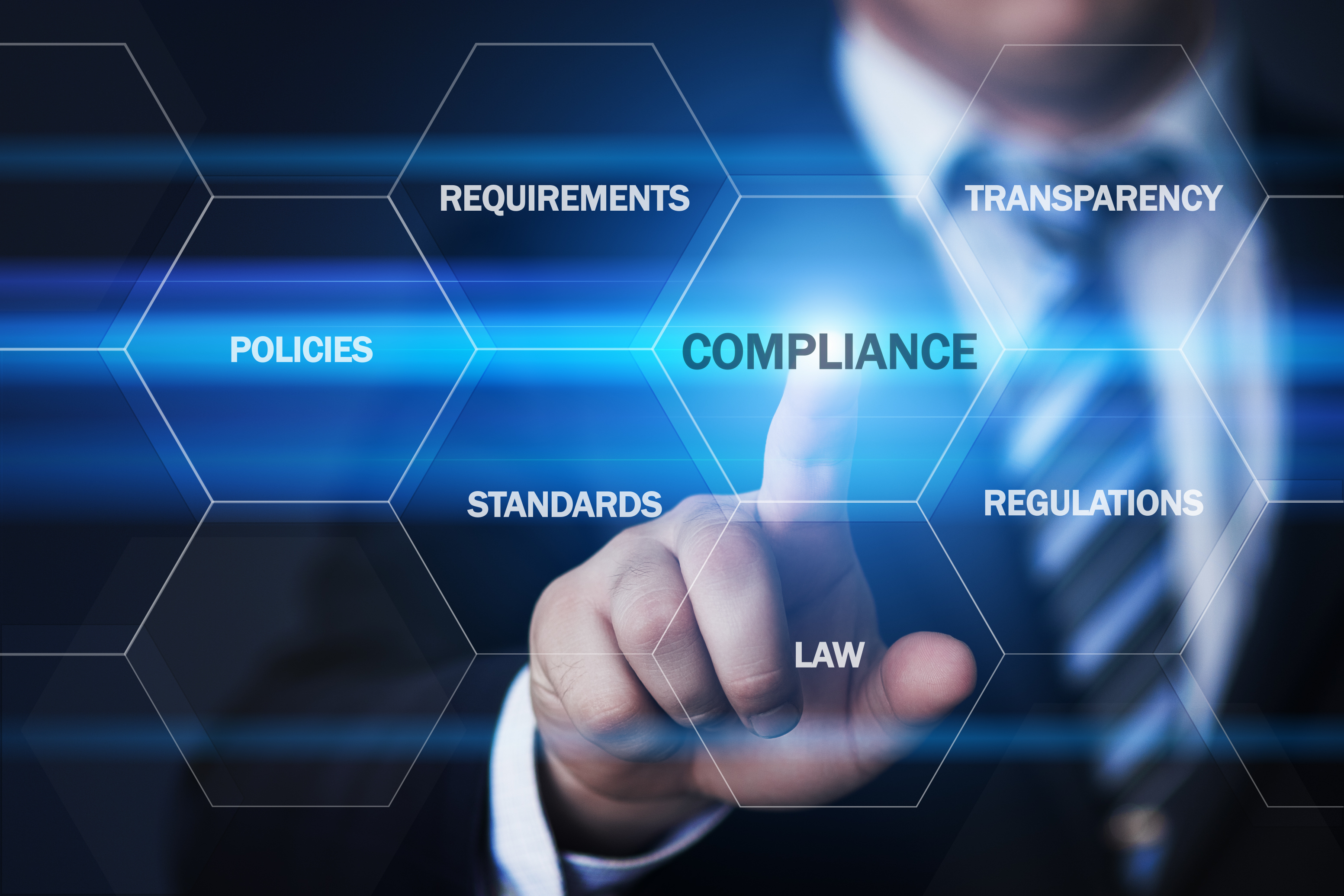
Installing AI Governance
AI Governance: Installing a framework in your business In the rapidly evolving ....
-min.jpg)
Ensuring Ethical AI: The Value of Third-Party Assessments
In the rapidly evolving landscape of artificial intelligence, organizations face....